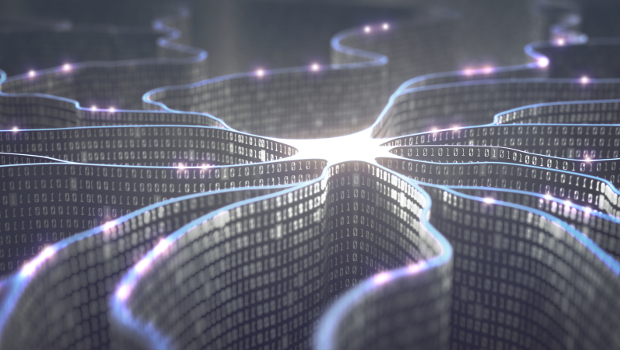
The real reality of artificial intelligence
Artificial intelligence is a somewhat vexing topic.
It is a headline grabber that allows the lazy headline writer to follow the formula “AI will revolutionise…” insert as appropriate. Or rather, as often as not, something inappropriate.
We have heard that it will have various sections of the workforce out of a job, changing the profession of lorry driver, legal secretary, even chef, if some of the speculation is to be believed.
But, so far, and indeed, for the foreseeable future, AI is unlikely to do these things.
“The cold reality is that with the current silicon-based machines, we are unlikely to ever be able to effectively emulate the human brain in all its complexity and subtlety of operation”
Certainly, driverless cars are developing at pace, but our perception of what artificial intelligence actually is currently, and our romanticised notions of it, are still divergent to say the least.
Decisions
Currently, AI is really just about making decisions. Whether it is an interactive voice system that can interpret your spoken wishes and direct your call, or it is a self-optimising application responding to certain measured aspects of performance, the systems usually have fairly clearly defined parameters and boundaries in which to operate, and even then within a narrowly defined scope.
Take for example, the big AI win lately being the Google DeepMind project that defeated a human champion at the Chinese game Go.
This was not like the famous IBM computer that beat the chess grand master Kasparov. For a start, Go has so many permutations as to be near infinite in its combinations. So brute force calculation was never going to work. Also, top Go players often report that some of their moves are made purely by intuition, from years of experience in play. The Google AI was allowed to learn the game from hundreds of thousands of examples of previously played games, and use its learning algorithms to reason out its play as it went.
Don’t get me wrong, the feat of being able to discern success and then learn the skills is still truly impressive, but it is within the narrow confines of a clearly defined, and simple environment, a board game – albeit one with infinite permutations. But it was not one with an element of the chaotic, say where weather might have played apart.
General purpose
Now the people behind the Google AI are working hard to make it a more general purpose system, but the reality is that it still needs vast amounts of data, on even the most simple of concepts, to succeed.
Despite this, there are any number of applications for such an AI. For example, in gene research, pharmaceuticals, chemistry, and more, such an AI could potentially speed up research by modelling and manipulating faster and more efficiently than physical experimentation. This could lead to more effective drugs with less side effects, chemicals that are potentially cleaner or more biodegradable than current versions, or those magic bacteria that might eat plastics, clean up nasty spills or eradicate a pathogen or harmful bacteria.
However, as yet, AI has fundamental problems, namely with perception. Seeing, and more accurately, observing is still an issue for AI. For computers to be able to look at a picture, or a scene, and discern exactly what is in that vista, is still some way away. While it is improving, it is still a long way away.
Processing
Facial recognition has come on in leaps and bounds, and there are companies that are offering solutions whereby ticketless entry to the likes of sports stadia are now a realistic proposition, with all the implications for both public order and privacy that entails. And yet, it is because they have been taught to find the patterns of faces, with a few critical parameters and work from there. We are not yet, nor are likely to be soon, where natural language processing (NLP) would allow a human to tell a general purpose AI to find all the faces in a video stream and then compare them with a known database to find a specific person, without telling it also what a face is and what it comprises.
Another critical element of this, and one which will be accelerated by the use of neural networks in AI, is that we tend to model AI systems on the human brain.
However, as was pointed out by an AI researcher and programmer Alex Champandard (see the News section of December 2018 TechPro), current technology is not capable of completely replicating the number of neurons in the human brain. Therefore, anything seeking to emulate it must be reduced in some way. Champandard asked how does one decide what to remove, what to leave out? Does that affect the outcome of the emulation?
The sad fact is, that we still do not fully understand our own brains. An old piece of graffiti from a pub I frequented as a student read “if the human brain was simple enough for us to understand it, we’d too simple to understand it”.
While that assertion is somewhat defeatist, it does highlight the fact that we still struggle understand at a fundamental level how our brains work. Therefore, to create a truly general purpose AI, we must make compromises due to our lack of understanding of our own general purpose intelligence.
Insights
Even when we gain certain insights into how our brain works, often through those recovering from injury, or those that experience conditions such as synaesthesia, those discoveries tend to result in more questions to ask than those answered.
The cold reality is that with the current silicon-based machines, we are unlikely to ever be able to effectively emulate the human brain in all its complexity and subtlety of operation.
More likely we will have to wait for the probably the second or even third generation of quantum computer before such emulations even approach nature. That said, as we develop our understanding through modelling the brains and nervous systems of more simple animals, our understanding grows. From nematode worms to fruit flies, simpler neural networks have yielded real insights into how apparently simple brains can produce complex behaviours and actions.
The upshot of all of this is that we stand now in terms of AI roughly where the clockwork automatons stood around the end of the nineteenth century in terms of that technology – at roughly the end of the development line. Only virtual modelling will allow us to go further, and even then, it may take a few leaps in capability to make it happen.
So for now, rest easy. A robot is unlikely to take your job any time soon, unless you are a driver, a secretary or legal researcher.
But watch out for breakthroughs that set new milestones. When a general purpose AI writes a symphony, paints a decent impressionist painting or writes a great screenplay, then it is time to sit up and take notice. For now, it would be good if they could simply do those things we find a drudge, a bore or a danger.
Subscribers 0
Fans 0
Followers 0
Followers